Many of our blogs have focused on unleashing the power of the edge by shifting decision making from the cloud to the field. They have focused on what is possible, what is coming in the future, and how this can help businesses make faster and smarter decisions.
While there are some incredibly exciting innovations happening in these areas that are unlocking amazing technological capabilities, today I’m focusing on the return on investment in these technologies. Cool tech gets developers, tinkerers, and implementers fired up, but ROI is really what motivates companies to adopt new technologies—the dollars and cents of shifting decision making to the edge.
Reduce Storage Cost with Edge Decision Making
One of the areas I typically focus on when communicating the ROI of edge computing to organizations is storage. This is because it’s quite straightforward and easy to communicate, regardless of technical experience.
Let’s say you have a fleet of 45,000 vehicles and you need to make decisions about driver safety based on speed. You’ll want those decisions to be based on high resolution data, meaning taking an average speed every second rather than every minute, few minutes, or hour. That is 2.7 million data points per minute (45,000 x 60). Let’s assume each one of those records is 2KB— an estimate based on my experience in IoT integrations. That is 5.4GB a minute, 324GB an hour, 7.7TB a day, 231TB a month, and 2.7PB a year.
If you were to run all of this data through Amazon Redshift this could cost you as much as $694,510.08 a month or $8,334,120.96 a year.
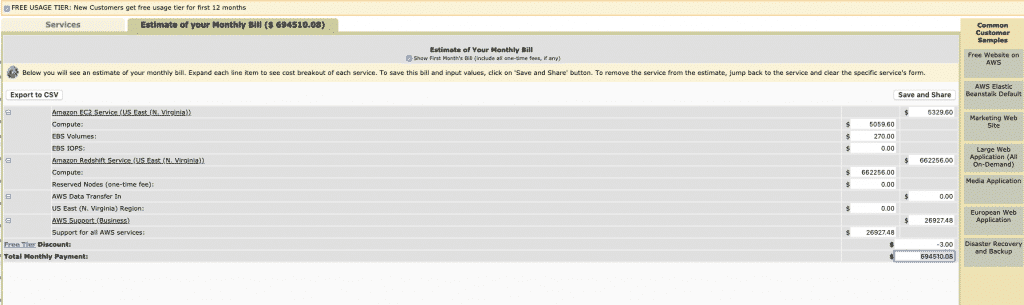
If this seems impossible, ask Uber or Lyft. At my former company we started out spending around $3k to $4k per month with AWS, which evolved into months of spending in excess of $70k. We were a tiny company so this represented an enormous portion of our burn.
Imagine that now you put computing platforms on each one of those 45,000 vehicles. Raspberry Pis are $35 and come with more than enough compute to do the job. That’s $1.57 million dollars in upfront hardware cost (though I suspect you could find a bulk discount in there somewhere). Remember that you own the hardware and therefore can depreciate it. You also obviously have the cost of extra storage, maintenance, and replacement, so let’s overestimate and add another 50%. Now you’re at $2.35 million over a 3 year period. That’s still significantly less than $24 million.
Worried about giving up cloud storage? Fair, but do you really need all that cloud? Let’s say that each one of those edge devices has 128GB of storage. That’s 5.76PB of storage (more than twice what you had on AWS), and you have a combined 180,000 cores and 45TB of RAM. This is all for a fixed cost of $2.35 million… not so crazy now, right?
Imagine that you could appropriately harness that compute with a distributed database. With a distributed supercomputer running on your fleet, instead of sending all that data to AWS, you only send the results of your calculations to AWS. You can use your local compute to take averages, do calculations, performs edge analytics, and make decisions. Let’s assume this results in sending one record every minute to AWS. That’s 3.85TB per month instead of 231TB, which drops your Redshift bill down to $14,435.35 per month or $172,220 per year. That’s pretty reasonable.
Your total cost of ownership over a 3 year period now reduces from $24 million to a little over $2 million. What about maintenance though? Without AWS you’ll need to hire your own people and manage a team. You could hire a team of ten IT folks at an average salary of $120,000 a year plus expenses, so around $150k a year per person. This would cost about $1.5 million a year or $4.5 million over 3 years. With this team of ten, your TCO of running a distributed computing platform would be $6.5 million, which is only 27% of the $24 million that it would cost to solely use a cloud platform. We also haven’t included depreciation or the fact that if you can stretch that hardware to last for five years it becomes a lot cheaper.
You may be questioning the value of data at high resolution and wondering if you really need it. What if you want to predict when your driver will need to get gas and ensure that they stop at the station with the cheapest fuel? What if you want to do video recognition for driver and vehicle safety to ensure that they pay attention to the road, or do predictive maintenance to predict that a vehicle is breaking down before it happens? The good news is that all of these things are possible with edge analytics. There were 4,237 fatal commercial vehicle crashes in the United States in 2017. This is an enormous loss of life and a huge economic cost. These vehicular accidents could be significantly reduced or prevented by utilizing data. However, the enormous cost of cloud computing is preventing companies from further investigating these options. The above thought experiment demonstrates that by utilizing an edge decision making platform, costs could be 89% less than the cost of leveraging the cloud for all decisioning making. We’re not done yet, this still doesn’t include other possible cost reductions such as communications…
The True Cost of Connectivity
There has been an enormous amount of hype around 5G. It will certainly solve some problems, but the rising costs of cloud computing is not one of them. If anything, 5G may exacerbate this problem due to an influx of data coming from numerous connected assets. Let’s take our above example and look at the communications cost of transmitting all that data. With a quick internet search the cheapest possible cost per GB of data transfer is around $1.00. If we take our example and consider that we are transferring 324GB an hour, thats $324 per hr, $7,776 per day and $2,838,240 per year. Our TOC for a cloud based solution over a 3 year period increases from $24 million to $32,514,720. The cost of connectivity is expensive, and sometimes even with unlimited budgets connectivity can be problematic. Consider an alternative network setup; an industrial facility with very high volume data sensors like Electrical Relays, Vibration Sensors, SCADA systems, and environmental controls. A slow network in a corporate environment may just cause people to be upset, but a slow network in an industrial environment can cause serious accidents. For example, a slow network causes a lag in temperature sensors from a boiler reporting high temperatures, which can cause detrimental damage or harm. These are real world concerns that we consider every day at HarperDB. Being able to make decisions directly on the edge means that this type of incident can be detected and alerted immediately with fewer points of failure, such as local area network failure. In summary, you can see how serious industrial accidents can be reduced or prevented for industries across the board with real time decisioning and alerting.
Distributed Computing is the Future
While centralized cloud can be awesome for many workloads, as more and more things become connected to the internet (IoT), it is critical that companies look at distributed computing environments that enable cloud capability on the edge in order to reduce cost, enhance decision making speed, improve security, and drive overall ROI in Industrial IoT.